티스토리 뷰
반응형
디지털 이미지를 기반으로 객체를 인식하거나 분류할 수 있는 이미지 분류 또는 컴퓨터 비전 시스템을 설계하는 작업인 인공 지능의 한 분야입니다.
응용 프로그램의 폭이 매우 넓기 때문에 인기 있는 분야입니다.
이미지 분류는 사진에서 특정 꽃을 인식하거나 의료 이미지를 정상 또는 질병으로 분류하는 것 등 다양한 문제에 사용할 수 있습니다.
이 글에서는 데이터 세트의 이미지를 분류하기 위해 컨볼루션 신경망을 만드는 데 Keras를 사용하는 방법에 대해 설명합니다.
이 글에서는 Keras에서 무료로 사용할 수 있는 사전 훈련된 모델 중 일부를 테스트하고
건물, 숲, 빙하, 산, 바다, 거리의 6가지 카테고리에 이미지를 이용해서 모델을 평가한다.
가장 먼저 해야 할 일은 Python 라이브러리에서 필요한 패키지를 가져오고 데이터를 학습할 수 있는 형태로 전처리 하는 것이다
import pandas as pd
import matplotlib as mat
import matplotlib.pyplot as plt
import numpy as np
import seaborn as sns
import random
import os
from numpy.random import seed
from sklearn.model_selection import train_test_split
from sklearn import metrics
from sklearn.metrics import accuracy_score
import tensorflow as tf
from tensorflow import keras
from tensorflow.keras import layers
from tensorflow.keras import callbacks
from tensorflow.keras.models import Model
from tensorflow.keras.preprocessing.image import ImageDataGenerator
import glob
import cv2
from tensorflow.random import set_seed
import warnings
from sklearn.preprocessing import OneHotEncoder
from sklearn.model_selection import train_test_split
from tensorflow.keras.models import Sequential, Model
from tensorflow.keras.layers import Dense, Flatten, Conv2D, MaxPool2D, GlobalAveragePooling2D, Dropout
from tensorflow.keras.applications.xception import Xception
from tensorflow.keras.optimizers import Adam
from sklearn.metrics import classification_report, confusion_matrix
또한 몇 가지 상수와 설정을 정의해야 합니다.
pd.options.display.max_colwidth = 100
seed(42)
random.seed(42)
os.environ['PYTHONHASHSEED'] = str(42)
os.environ['TF_DETERMINISTIC_OPS'] = '1'
set_seed(42)
warnings.filterwarnings('ignore')
IMG_SIZE = 150
SEED = 42
파일에 액세스하여 모든 디렉토리에서 이미지 파일을 가져오기 위해 다음을 사용했습니다.
main_path = "/content/drive/MyDrive/ic_project"
train_path = os.path.join(main_path,"train")
train_buildings = glob.glob(train_path+"/buildings/*.jpg")
train_forest = glob.glob(train_path+"/forest/*.jpg")
train_glacier = glob.glob(train_path+"/glacier/*.jpg")
train_mountain = glob.glob(train_path+"/mountain/*.jpg")
train_sea = glob.glob(train_path+"/forest/*.jpg")
train_street = glob.glob(train_path+"/street/*.jpg")
그런 다음 다른 디렉토리의 모든 이미지를 하나의 단일 목록에 결합합니다. 사실, 나열하자면 데이터 프레임을 테스트하고 훈련합니다.
#Train list:
train_list = [x for x in train_buildings]
train_list.extend([x for x in train_forest])
train_list.extend([x for x in train_glacier])
train_list.extend([x for x in train_mountain])
train_list.extend([x for x in train_sea])
train_list.extend([x for x in train_street])
df_train = pd.DataFrame(np.concatenate([
['0']*len(train_buildings),
['1']*len(train_forest),
['2']*len(train_glacier),
['3']*len(train_mountain),
['4']*len(train_sea),
['5']*len(train_street)]),
columns = ['label'])
df_train['image'] = [x for x in train_list]
획득한 목록을 확인할 수 있습니다.
df_train
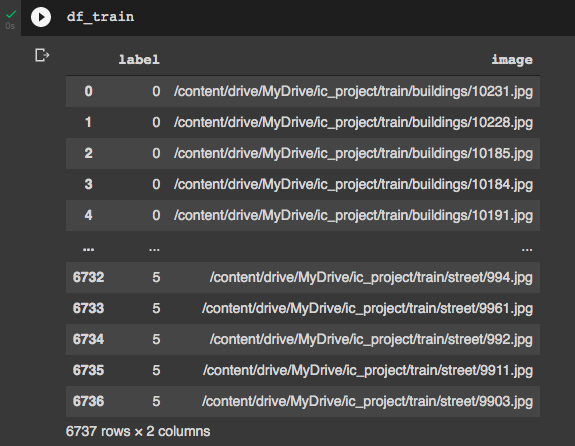
반응형
'머신러닝 & 딥러닝' 카테고리의 다른 글
[실습-4] 다중 클래스 이미지 딥러닝 예측 모델 기본 구조 (0) | 2022.08.21 |
---|---|
opencv-python 패키지 설치 및 이미지 불러오기 (0) | 2022.08.20 |
Tensorflow Keras - CNN ImageDataGenerator 활용하기 (0) | 2022.08.12 |
cnn 모델을 이용한 꽃 종류를 예측하기 (0) | 2022.08.12 |
딥러닝 시계열 분석 기본 방법 (0) | 2022.08.11 |
댓글